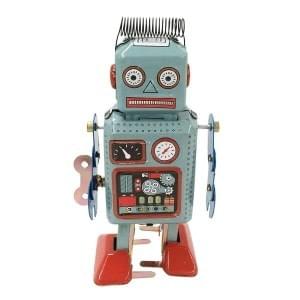
Parlons Futur : "Pas le temps de faire une veille approfondie pour anticiper le futur? Pas de soucis, je la fais pour vous! Pour vous faire gagner du temps, voici une sélection de news du futur dont j'ai supprimé le blabla pour ne garder que la substance, restructurée en liste de points, parfois assortis de mes précisions."
Les articles à suivre que j'ai donc "raccourcis" et structurés en "bullet points":
- 1. Qu'est-ce qu'est le Machine Learning ? (dec 2017, 1650 mots raccourcis à 430, insign.fr)
- 2. Even if genes affect intelligence, we can’t engineer cleverness (dec 2017, 1200 mots raccourcis à 240, aeon.co)
- 3. New study about the 20 emerging issues in bioengineering (dec 2017, 1000+ mots raccourcis à 600, Singularityhub.com)
- 4. China’s CCTV System Tracked Down a Reporter in Only Seven Minutes (dec 2017, 400 mots raccourcis à 90, futurism.com)
- 5. Une première : cette entreprise entend tester ses voitures en autonome en réalité virtuelle et pas que dans la "vraie vie" (dec 2017, 1200 mots raccourcis à 180, Singularityhub.com)
- 6. An AI-Equipped Microscope Can Diagnose Deadly Blood Infections (dec 2017, 400 mots raccourcis à 100, futurism.com)
- 7. New lift technology is reshaping cities (dec 2017, 2600 mots raccourcis à 600, The Economist)
- 8. Des chercheurs trouve la faille d'une IA de Google, lui faisant confondre sur une photo chat et guacamole (dec 2017, 950 mots raccourcis à 350, wired.com)
- 9. Artificial wombs (utérus) are coming. They could completely change the debate over abortion. (août 2017, 2300 mots raccourcis en 650, vox.com)
- 10. The data that transformed AI research—and possibly the world (juillet 2017, 2640 mots raccourcis en 600 , qz.com)
- 11. Meet Baby Emma. She Was Frozen as an Embryo for 24 Years. (déc 2017, 570 mots raccourcis à 125, futurism.com)
- 12. As Artificial Intelligence Advances, Here Are Five Tough Projects for 2018 (dec 2017, 1350 mots raccourcis à 600, wired.com)
- 13. "Reality 2.0": the new concept that merges Virtual and Augmented Reality (dec 2017, 1830 mots raccourcis à 600, singularityhub.com)
- 14. Deep Learning Achievements Over the Past Year (dec 2017, 3650 mots raccourcis à 740, blog.statsbot.co)
- 15. The evolution of machine learning (août 2017, 1330 mots raccourcis à 225, techcrunch.com)
- 16. AI Software Learns to Make AI Software (jan 2017, 570 mots raccourcis à 260, technologyreview.com)
- 17. Google’s AutoML lets you train custom machine learning models without having to code (jan 2017, 465 mots raccourcis à 300, techcrunch.com)
[Pour info: j'ai aussi créé un podcast, tapez simplement Parlons Futur dans votre appli de podcast préférée (si vous n'en avez pas je recommande Podcast Republic). Pratique pour se brieffer sur le futur "on the go", à vous de choisir la vitesse et de faire avance rapide quand je vous saoule! Je débute, je vous remercie par avance pour votre indulgence, et vos retours, conseils, remarques sont les bienvenus ! :)]
Voir tous mes derniers tweets : https://twitter.com/thomasjestin
_______
Et maintenant à table :
1. Qu'est-ce qu'est le Machine Learning ? (dec 2017, 1650 mots raccourcis à 430, insign.fr)
- Machine Learning = Learning. Afin de pouvoir décider, la machine doit apprendre.
- C'est ce qui distingue le ML de l'Intelligence Artificielle traditionnelle où il faut tout coder "à la main", en prévoyant tous les cas de figure.
- A partir de données « connues », on va entraîner le modèle par itérations. A chaque itération la qualité des prévisions obtenues est testée (sur un jeu de données « témoin »). Si l’erreur de prévision est importante, on fait varier certains paramètres de l’algorithme. Les itérations s’arrêtent quand l’erreur de prévision ne baisse plus et le modèle est implémenté en production ; la machine a appris des « patterns » et est en mesure de détecter très efficacement les cas similaires.
- Alphabet (maison mère de Google) a créé une solution de Machine Learning au travers d’une librairie logicielle Open Source TensorFlowTM, couplée à une solution matérielle ; ses propres cartes électroniques optimisées pour le traitement des données soumises au Machine Learning (TPU : TensorFlowTM Processing Units) accessibles en Cloud.
- Le Machine Learning, ce n’est ni de la voyance, ni de la divination. Sous cette appellation se trouvent des méthodes scientifiques qui s’appuient sur des modèles mathématiques, parfois empruntés à la biologie3. Ces algorithmes analysent des données pour réaliser des identifications et des classements.
- Ce sujet fait le buzz, or ces algorithmes sont connus depuis plusieurs dizaines d’années et vous en faites déjà l’expérience dans votre quotidien :
- Estimation du prix d’une maison en fonction de ses caractéristiques sur un site immobilier ;
- Calcul du risque d’accident et des tarifs de votre assurance ;
- Filtrage des e-mails « spam » de votre logiciel ou fournisseur.
- Ce qui change cependant très rapidement, c’est l’extension de ces traitements à de nouvelles données (textes, audio, photos, localisation, vidéo, capteurs IoT) et la disponibilité d’immenses puissances de stockage et de calcul pour proposer de nouveaux usages moins verticaux, moins « industriels ».
- Cette puissance de calcul disponible (dans le Cloud et avec des coûts qui baissent) et l’abondance de données renforcent les capacités et la précision d’un modèle pour :
- Définir le contenu d’une image sur le web ou prise en photo avec votre smartphone : distinction (chien ou chat), identification (café ou restaurant), classement (nombre, ordre, plans), dans le e-commerce c'est la possibilité de soumettre n’importe quelle photo pour trouver les vêtements sur l'app par exemple
- Déterminer le risque d’occurrence d’une panne sur une machine en fonction de paramètres exogènes (météo, événements, usagers, ...) ; très utile désormais avec l’essor des objets connectés pour incarner vos services auprès de vos clients ;
- Prédire le prochain mot sur un clavier en fonction du contexte (interlocuteur et historique de la relation, émotion mesurée, ...).
______
2. Even if genes affect intelligence, we can’t engineer cleverness (dec 2017, 1200 mots raccourcis à 240, aeon.co)
- By computational biologist Jim Kozubek based in Cambridge, Massachusetts
- A paper published in Nature Genetics in 2017 reported that, after analysing tens of thousands of genomes, scientists had tied 52 genes to human intelligence, though no single variant contributed more than a tiny fraction of a single percentage point to intelligence.
- "there’s a long way to go’ before scientists can actually predict intelligence using genetics."
- Even so, it is easy to imagine social impacts that are unsettling: students stapling their genome sequencing results to their college applications; potential employers mining genetic data for candidates; in-vitro fertilisation clinics promising IQ boosts using powerful new tools such as the genome-editing system CRISPR-Cas9.
- Some philosophers are starting to talk about : ‘genetic neglect’, suggesting that if we don’t use genetic engineering or cognitive enhancement to improve our children when we can, it’s a form of abuse.
- As it turns out, genes contribute to intelligence, but only broadly, and with subtle effect. Genes interact in complex relationships to create neural systems that might be impossible to reverse-engineer.
- Importantly, genetics research is not about to diagnose, treat or eradicate mental disorders, or be used to explain the complex interactions that give rise to intelligence. We won’t engineer superhumans any time soon.
- Importantly, we have known for a long time that 30,000 genes cannot determine the organisation of the brain’s 100 trillion synaptic connections, pointing to the irrefutable reality that intelligence is, to an extent, forged through adversity and the stress of developing a brain.
______
3. New study about the 20 emerging issues in bioengineering (dec 2017, 1000+ mots raccourcis à 600, Singularityhub.com)
- CRISPR-Cas9, the genome editing technique discovered in 2014, provides an opportunity to solve problems in food supply, disease, genetics, and—the most tantalizing and forbidden of prospects—modifying the human genome. Doing so would make us better, faster, stronger, more resilient, and more intelligent: it’s a chance to engineer ourselves at a faster rate than natural selection could ever dream.
- CRISPR may make it possible to create the bioweapons carefully safeguarded by the US and Russian governments, such as smallpox, or to take an existing disease, like Ebola, and modify it into an epidemiologist’s worst nightmare.
- A new study combining researchers from the US and the UK, published recently in eLifeSciences, gives an expert perspective on 20 emerging issues in bioengineering.
- The researchers sorted the 20 developments into different time horizons: the next 5 years, the next 10 years, and more than a decade away.
- In the next 5 years, they anticipate breakthroughs in artificial photosynthesis.
- As plants can turn carbon dioxide into fuel, artificial photosynthesis could be crucial for the energy crisis and to tackle climate change. Recent studies have shown that artificial photosynthesis can reduce CO2 more efficiently than plants and can convert it into methanol for fuel.
- Enhancing natural photosynthesis through genetic modification may prove to be the answer to feeding the world, as for instance there's a gene that could be engineered into rice and boost rice yields by up to 50 percent. Since rice provides hundreds of millions with most of their calories, it’s a huge potential development.
- The researchers also expect 2 major debates to begin in earnest over the next 5 years.
- The first is about the ethics of "gene drives", which can “force” a population to inherit new characteristics. For insects like mosquitos, these genes can spread very rapidly, and people have suggested using them to render mosquitos infertile. At the same time, they could wreak havoc on ecosystems and may have unintended consequences. Is the solution an outright ban on the use of gene drives or can we find clever ways to control these gene drives, and “de-activate” them before they spread too far, or after a certain number of generations?
- The big debate will kick into gear over the next five years: how comfortable are we with editing the human genome? The researchers note that our capacity to edit the human genome has already surpassed our understanding of the functions of these genes. Perhaps careful editing will allow us to perform experiments that will unlock a greater understanding of our own DNA; already, in mice, we can knock out conditions like Huntington’s disease.
- After 5 years and before 10 years, researchers worry about ever more sophisticated bioengineering technologies. Perhaps, in five to ten years, we will be able to construct whole replacement organs through tissue engineering. They note that in the last few years, “Tissue engineers have already built or grown transplantable bladders, hip joints, vaginas, windpipes, veins, arteries, ears, skin, the meniscus of the knee, and patches for damaged hearts.”
- But it’s unlikely to be cheap: could this exacerbate the healthcare gaps that already exist in society, with the super-rich able to afford to replace their organs and extend their lifespans while the rest of us are doomed to death and taxes?
- The far-reaching ability of these techniques will impact drug manufacturing in unprecedented ways. Vaccines are a textbook example. Currently, many vaccines are produced using hen’s eggs as incubators, the same technique that’s been used for 70 years. As expected, there are limitations to such an old method; the most important strains of a virus must be predicted months in advance because vaccine production takes several months to complete.
- DARPA—funders of the Internet, self-driving cars, and cool disaster robots—awarded a grant to a company that could produce ten million flu vaccines within a month. If we’re in a race against the next pandemic—which could kill millions, as the Spanish influenza did—then it almost seems a moral imperative to develop this technology.
- Yet as it becomes accessible by more and more people, there are other risks. Illegal drugs could be manufactured more efficiently through bioengineering. Far worse is the prospect of a bioengineered super-virus, created by accident or maliciously.
- In the next 5 years, they anticipate breakthroughs in artificial photosynthesis.
______
4. China’s CCTV System Tracked Down a Reporter in Only Seven Minutes (dec 2017, 400 mots raccourcis à 90, futurism.com)
- There are 170 million CCTV cameras in place across China, with 400 million more are set to be installed by 2020. Boasting the world’s largest CCTV monitoring system, the country is also investing in artificial intelligence (AI) and facial recognition technologies.
- In a demonstration of technological prowess, Chinese officials located and apprehended BBC reporter John Sudworth only seven minutes after his image was “flagged to authorities.”
- This wasn’t a real arrest, however. It was merely an exercise to show the power of both the nation’s CCTV cameras and advanced facial recognition technology.
______
5. Une première : cette entreprise entend tester ses voitures en autonome en réalité virtuelle et pas que dans la "vraie vie" (dec 2017, 1200 mots raccourcis à 180, Singularityhub.com)
- Waymo recently announced its fleet has now driven four million miles autonomously. That’s a lot of miles, and hard to compete with. But AImotive isn’t trying to compete, at least not by logging more real-life test miles. Instead, the company is doing 90 percent of its testing in virtual reality. “This is what truly differentiates us from competitors,” Kishonti said.
- the 3 main benefits of VR testing, it can simulate scenarios :
- too dangerous for the real world (such as hitting something),
- too costly (not every company has Waymo’s funds to run hundreds of cars on real roads),
- or too time-consuming (like waiting for rain, snow, or other weather conditions to occur naturally and repeatedly).
- “Real-world traffic testing is very skewed towards the boring miles,” he said. “What we want to do is test all the cases that are hard to solve.”
- In one simulation, a furry kangaroo suddenly hopped across one screen. “Volvo had an issue in Australia,” Kishonti explained. “A kangaroo’s movement is different than other animals since it hops instead of running.”
- AImotive is currently testing around 1,000 simulated scenarios every night, with a steadily-rising curve of successful tests.
______
6. An AI-Equipped Microscope Can Diagnose Deadly Blood Infections (dec 2017, 400 mots raccourcis à 100, futurism.com)
- A team of researchers from a Harvard University teaching hospital have developed a microscope equipped with AI that can diagnose blood infections. The AI was able to categorize 93 percent of samples without human help.
- Artificial intelligence is still in the early stages of its relationship with medicine, but great strides are being made: not long ago, a Chinese robot was even able to pass a medical licensing exam. We may not know the full capacity of what the medical field will look like with AI and robots lending a hand but the life-saving potential of non-human helpers is certainly exciting.
______
7. New lift technology is reshaping cities (dec 2017, 2600 mots raccourcis à 600, The Economist)
- Around 1bn people take one of the world’s 14m lifts every day; they take twice as many lift journeys in a day as people take flights in a year.
- Hoisting equipment of one sort or another has been in use for millennia. The Colosseum in Rome had 24 lifts powered by slaves.
- Louis XV installed a counterweight lift to his private chambers in Versailles in 1743.
- The electric motor revolutionised the lift industry. Otis’s original steam-powered lift climbed at 0.2 meter/s.
- The electrified lifts in the first steel-framed building to top 50 floors, the 241-metre Woolworth Building, which opened in 1913, were more than 10 times faster.
- By 1933, those in the 381-metre Empire State Building travelled at 6 meter/s, as fast as many modern lifts.
- Before the 20th century people prized proximity to the pavement. The first floor, above the hubbub of the street but conveniently accessed by a single flight of stairs, was the floor most sought after—the piano nobile or bel étage. Anything above the second floor was typically reserved for servants. In hotels and tenements, standards and prices fell with altitude.Top floors were considered a public-health risk. The strain of tackling so many stairs, the difficulty of getting outside in the fresh air and the trapped heat of summer played a part in this. It may be no coincidence that the garrett (mansarde) was home to consumptive artists.
- The lift not only made much higher floors possible, it gave them a new status and glamour. Rents began to rise, not fall, with height. The penthouse—a word that took its modern meaning in the 1920s—became a status symbol. From the Equitable Life Building onwards, top executives took to the top floors. Altitude was eminence, farsightedness, elevation—power.
- By the 1970s lift engineering was a pretty mature industry, and started to consolidate and globalise
- 4 giants : Kone and Thyssenkrupp, along with the Swiss firm Schindler, bought up rival firms to join Otis (now a division of United Technologies) as worldwide brands.
- Between them the big 4 now account for around 2/3 of the global market;
- Hitachi and Mitsubishi Electric of Japan take quite a lot of the rest.
- There is as yet no Chinese lift giant—perhaps because the industry relies as much on its ability to provide services on a global scale as on its mechanical engineering prowess.
- 50% of the big 4's annual revenues of €36bn come from services
- In 2000 some 40,000 new lifts were installed in China. By 2016 the number was 600,000—almost 3/4 of the 825,000 sold worldwide.
- More than 100 buildings round the world are over 300 metres; almost all of them were built since 2000, and nearly half of them in China
- China is home to 2/3 of the 128 buildings over 200 metres completed in 2016
- Study : after 28 seconds waiting, would-be passengers start to get irritated
- Lifts typically travel at around 8-9 meter/s
- Mitsubishi’s lifts in the Shanghai Tower reach 20m/s (45 miles per hour)
- When the Jeddah Tower in Saudi Arabia, the world’s first 1km building, opens in 2020 it will boast a 660-metre lift made possible by UltraRope; the company thinks doing a whole kilometre should be feasible, if anyone wants to.
- A development being tested aims at doing away with the cable altogether.
- Thyssenkrupp has harnessed high-speed rail technology to create Multi, a system held in place and accelerated by electromagnetic forces like those used for magnetic-levitation trains.
- For the anecdote : One of the first people to look into it was a PhD student in Manchester in the 1970s, Haider al-Abadi, who is now prime minister of Iraq.
- The absence of cables will also allow lifts to move laterally, as well as vertically, making the whole system more like a railway. Lift shafts will be able to fork and rejoin to allow overtaking; descending lifts could sidestep ascending ones.
- Transport hubs could house lifts serving a range of local buildings, moving first horizontally, then vertically.
______
8. Des chercheurs trouve la faille d'une IA de Google, lui faisant confondre sur une photo chat et guacamole (dec 2017, 950 mots raccourcis à 350, wired.com)
- Algorithms, unlike humans, are susceptible to a specific type of problem called an “adversarial example.” These are specially designed optical illusions that fool computers into doing things like mistake a picture of a panda for one of a gibbon. They can be images, sounds, or paragraphs of text. Think of them as hallucinations for algorithms.
- an adversarial example could thwart the AI system that controls a self-driving car, for instance, causing it to mistake a stop sign for a speed limit one. They’ve already been used to beat other kinds of algorithms, like spam filters.
- Those adversarial examples are also much easier to create than was previously understood
- team from MIT reliably fooled Google’s Cloud Vision API, a machine learning algorithm used in the real world today.
- in this latest study, the MIT researchers did their work under “black box” conditions, thatis, without any level of insight into the target algorithm
- they fooled Google’s algorithm into believing a photo of a row of machine guns was instead a picture of a helicopter, merely by slightly tweaking the pixels in the photo. To the human eye, the two images look identical. The indiscernible difference only fools the machine.
- The researchers randomly generated their labels; in the rifle example, the classifier “helicopter” could just as easily have been “antelope.” They wanted to prove that their system worked, no matter what labels were chosen. “We can do this given anything. There’s no bias, we didn’t choose what was easy,”
- MIT’s latest work demonstrates that attackers could potentially create adversarial examples that can trip up commercial AI systems. Google is generally considered to have one of the best security teams in the world, but one of its most futuristic products is subject to hallucinations.
- Researchers have essentially created artificially intelligent systems that “think” in different ways than humans do, and no one is quite sure how they work.
- “I can show you two images that look exactly the same to you, and yet the classifier thinks one is a cat and one is a guacamole with 99.99 percent probability.”
- These kinds of attacks could one day be used to, say, dupe a luggage-scanning algorithm into thinking an explosive is a teddy bear, or a facial-recognition system into thinking the wrong person committed a crime.
______
9. Artificial wombs (utérus) are coming. They could completely change the debate over abortion. (août 2017, 2300 mots raccourcis en 650, vox.com)
- Tribune de Glenn Cohen, professor at Harvard Law School
- The research remains preliminary, but in April 2017 a group of scientists at the Children’s Hospital of Philadelphia announced amazing advances in artificial womb technologies. The authors explained how they had successfully sustained significantly premature lambs for four weeks in an artificial womb
- This enabled the lambs to develop in a way very similar to lambs that had developed in their mothers’ wombs. Indeed, the oldest lamb — more than a year old at the time the paper was published — appeared to be completely normal.
- The technology included placing the premature lambs in a “biobag” containing a bath of simulated amniotic fluid, regularly replenished, with an oxygenator circuit connected to the lamb via the umbilical cord.
- The lambs were at a stage of development comparable to that of a 22- to 24-week-old human fetus. Babies born at that stage of gestation have very high mortality rates —roughly 70 percent at 22 weeks — and almost all who survive have long-term health problems. The immediate hope is that artificial wombs could raise the survival rate of human fetuses and improve their lifelong health substantially.
- If — and it is a big “if” — artificial wombs were to become available for human fetuses, we face the following question: Could anti-abortion laws require pregnant women whose fetuses are not yet viable to transfer the fetus to a nurturing site outside the body, possibly by way of minimally invasive surgery? The right to abortion would thereby be restricted.
- the constitutional right to abortion in America actually amounts to a conjunction of three separate but overlapping “rights not to procreate.”
- First, there is a right not to be a gestational parent: That is, a woman has the right to stop gestating, or carrying a fetus to term.
- Second, there is a right not to be a legal parent: The law cannot force on a woman, against her wishes, the legal duties of parenthood.
- Finally, a right not to be a genetic parent — for there to be no child that comes into being that is her genetic offspring.
- Typically, when a woman has an abortion, she is able to prevent all three kinds of parentage: She stops gestating the child, there is no child that bears her genetic code that comes into existence, and (therefore) there is no child the law recognizes as her child.
- Now what about a law decreeing that although abortion is available up to that 18-week threshold, once transfer to an artificial womb is possible, a woman who wants to stop gestating cannot abort ?
- She can either continue her pregnancy or transfer the fetus to the artificial womb.
- This would effectively preserve her right not to be a gestational parent — as she can stop gestating by transfer to the artificial womb — but not her right not to be a genetic parent.
- This scenario raises a crucial question: Do those who support abortion rights support :
- the right of women to control only whether they gestate
- or, additionally, a right to terminate a fetus whether or not gestation is involved?
- Certainly, the rhetoric of abortion rights in America focuses on avoiding unwanted gestation — think of the slogan “my body, my choice.”
- In a world of artificial wombs, a woman might have a right to stop gestating (to transfer the fetus out of the body to an artificial womb provided it's via a “minimally invasive surgery”) but not a right to terminate the fetus as well.
- Distinguishing between ceasing gestation and terminating a fetus could have some important implications for paternal rights.
- Confronted with the argument that transfer to an artificial womb could be made mandatory, a different strategy might be to stand up and defend abortion as a right not to be a genetic parent — full stop.
- It seems an unalloyed good that prematurely born fetuses may eventually have a greatly improved chance to live and thrive. But welcome new advances also sometimes raise new questions. Here, these laudable medical advances also reopen a host of complicated questions about one of the most hotly contested issues in politics, law, and ethics.
_______
10. The data that transformed AI research—and possibly the world (juillet 2017, 2640 mots raccourcis en 600 , qz.com)
- ImageNet, originally published in 2009 , is a dataset of pictures that quickly evolved into an annual competition to see which algorithms could identify objects in the dataset’s images with the lowest error rate. Many see it as the catalyst for the AI boom the world is experiencing today.
- 2017 was the final year of the competition. In just seven years, the winning accuracy in classifying objects in the dataset rose from 71.8% to 97.3%, surpassing human abilities and effectively proving that bigger data leads to better decisions.
- Original dataset took two and a half years to complete. It consisted of 3.2 million labelled images, separated into 5,247 categories, sorted into 12 subtrees like “mammal,” “vehicle,” and “furniture.”
- As the competition continued in 2011 and into 2012, it soon became a benchmark for how well image classification algorithms fared against the most complex visual dataset assembled at the time.
- Two years after the first ImageNet competition, in 2012, something even bigger happened. Indeed, if the artificial intelligence boom we see today could be attributed to a single event, it would be the announcement of the 2012 ImageNet challenge results.
- Geoffrey Hinton, Ilya Sutskever, and Alex Krizhevsky from the University of Toronto submitted a "deep neural network architecture called AlexNet—still used in research to this day—which beat the field by a whopping 10.8 percentage point margin, which was 41% better than the next best.
- ImageNet couldn’t come at a better time for Hinton and his two students. Hinton had been working on artificial neural networks since the 1980s, and while some like Yann LeCun had been able to work the technology into ATM check readers through the influence of Bell Labs, Hinton’s research hadn’t found that kind of home. A few years earlier, research from graphics-card manufacturer Nvidia had made these networks process faster, but still not better than other techniques.
- Hinton and his team had demonstrated that their networks could perform smaller tasks on smaller datasets, like handwriting detection, but they needed much more data to be useful in the real world.
- Today, these neural networks are everywhere—Facebook, where LeCun is director of AI research, uses them to tag your photos; self-driving cars are using them to detect objects; basically anything that knows what’s in a image or video uses them. They can tell what’s in an image by finding patterns between pixels on ascending levels of abstraction, using thousands to millions of tiny computations on each level. New images are put through the process to match their patterns to learned patterns. Hinton had been pushing his colleagues to take them seriously for decades, but now he had proof that they could beat other state of the art techniques.
- Today, many consider ImageNet solved—the error rate is incredibly low at around 2%. But that’s for classification, or identifying which object is in an image. This doesn’t mean an algorithm knows the properties of that object, where it comes from, what it’s used for, who made it, or how it interacts with its surroundings. In short, it doesn’t actually understand what it’s seeing.
- This is mirrored in speech recognition, and even in much of natural language processing. While our AI today is fantastic at knowing what things are, understanding these objects in the context of the world is next. How AI researchers will get there is still unclear.
- In 2016, Google released the Open Images database, containing 9 million images in 6,000 categories. Google recently updated the dataset to include labels for where specific objects were located in each image.
- (comme quoi les GAFAM ne gardent pas tous leurs trouvailles pour eux, c'est d'ailleurs à cette condition qu'ils parviennent à faire venir et garder les meilleurs chercheurs comme Yann LeCun, le Français de Facebook)
______
11. Meet Baby Emma. She Was Frozen as an Embryo for 24 Years. (déc 2017, 570 mots raccourcis à 125, futurism.com)
- In November 2017, a baby was successfully born from an embryo frozen for 24 years — the longest period a viable embryo has ever been stored.
- This means for instance that those with cancer who anticipate losing fertility after chemotherapy treatments could store a healthy embryo until they are ready to be a parent.
- Earlier in 2017, a woman in the U.S successfully gave birth after receiving a uterus transplant. This promising development shows that women with severe medical complications, who are born without a uterus, or who are transgender could still potentially give birth.
- In another fertility marvel, a woman successfully gave birth thanks to an ovary that was removed pre-puberty. There is even the promise of 3D-printed ovaries, which successfully allowed infertile mice to give birth.
______
12. As Artificial Intelligence Advances, Here Are Five Tough Projects for 2018 (dec 2017, 1350 mots raccourcis à 600, wired.com)
- Understanding the meaning of words
- There is work to give machines some common sense and basic understanding of the physical world that underpins our own thinking.
- Facebook researchers are trying to teach software to understand reality by watching video, for example.
- Google has been tinkering with software that tries to learn metaphors.
- The reality gap impeding the robot revolution
- Why are we not all surrounded by bustling mechanical helpers? Today’s robots lack the brains to match their sophisticated brawn (muscle).
- Getting a robot to do anything requires specific programming for a particular task.
- They can learn operations like grasping objects from repeated trials (and errors). But the process is relatively slow. One promising shortcut is to have robots train in virtual, simulated worlds, and then download that hard-won knowledge into physical robot bodies.
- Yet that approach is afflicted by the reality gap—a phrase describing how skills a robot learned in simulation do not always work when transferred to a machine in the physical world
- The reality gap is narrowing. In October, Google reported promising results in experiments where simulated and real robot arms learned to pick up diverse objects including tape dispensers, toys, and combs.
- Guarding against AI hacking
- Researchers showed this year that you can hide a secret trigger inside a machine-learning system that causes it to flip into evil mode at the sight of a particular signal. The team at NYU devised a street-sign recognition system that functioned normally—unless it saw a yellow Post-It. Attaching one of the sticky notes to a stop sign in Brooklyn caused the system to report the sign as a speed limit. The potential for such tricks might pose problems for self-driving cars.
- Using technology to manipulate people is inevitable as machine learning becomes easier to deploy, and more powerful. “You no longer need a room full of PhDs to do machine learning,” he said.
- The Russian disinformation campaign during the 2016 presidential election is a potential forerunner of AI-enhanced information war.
- One trick Hwang predicts could be particularly effective is using machine learning to generate fake video and audio.
- Graduating beyond boardgames
- Chess, shogi, and Go are complex but all have relatively simple rules and gameplay visible to both opponents. They are a good match for computers’ ability to rapidly spool through many possible future positions. But most situations and problems in life are not so neatly structured.
- That’s why DeepMind and Facebook both started working on the multiplayer videogame StarCraft in 2017. Neither have yet gotten very far. Right now, the best bots—built by amateurs—are no match for even moderately-skilled players.
- DeepMind researcher said in 2017 that his software now lacks the planning and memory capabilities needed to carefully assemble and command an army while anticipating and reacting to moves by opponents.
- Not coincidentally, those skills would also make software much better at helping with real-world tasks such as office work or real military operations.
- Teaching AI to distinguish right from wrong
- some people are working on techniques that can be used to audit the internal workings of AI systems, and ensure they make fair decisions when put to work in industries such as finance or healthcare.
- Google, Facebook, Microsoft, and others have begun talking about keeping AI on the right side of humanity, and are members of a new nonprofit called Partnership on AI that will research and try to shape the societal implications of AI.
- A philanthropic project called the Ethics and Governance of Artificial Intelligence Fund is supporting MIT, Harvard, and others to research AI and the public interest.
- A new research institute at NYU, AI Now, has a similar mission. In a recent report it called for governments to swear off using “black box” algorithms not open to public inspection in areas such as criminal justice or welfare.
______
13. "Reality 2.0": the new concept that merges Virtual and Augmented Reality (dec 2017, 1830 mots raccourcis à 600, singularityhub.com)
- Reality 2.0 is literally about replacing your visual perception of the world with a fully virtual 3D environment, but one that respects the constraints of the place where your are
- Imagine taking an exact laser scan of your current environment—your living room or office space, or any other thing—and using this to create a virtual 3D replica of everything around you. Now, re-create the room in VR and overlay the real world with the virtual experience. You are experiencing an upgradable version of reality in which you can modify the elements of your virtual world however you desire.
- Full control over your perception will let you do some pretty spectacular things, like replacing a random person with a famous actor in real-time, transforming your current room into a beach hut, re-experiencing any given moment that happened in this room, and much more.
- The ability to change parts of your environment
- it is all about changing aspects of the objects in your world, like the color or material it is made from. You could also replace the object itself, as long as you keep the basic geometry intact: My couch might as well be an ancient roman sofa in my perception
- The basic idea behind replacing objects is the adaptability of the human brain. One of the most interesting experiments in this area is the rubber hand experiment, where subjects started associating themselves with a rubber hand, even experiencing phantom pain, all because of the visual impression that the rubber hand is a part of their body.
- Changing objects or aspects of objects, like turning wood into stone, should be hugely compensated by the brain’s natural adaptability in an extended experience of the new environment.
- We will be able to create 3D recordings of any environment and play them back later. Imagine for a second that this kind of technology already existed a few decades ago. What a huge improvement it would be compared to staring at a burned out picture in a photo book.
- Why is it better than current VR?
- Currently, VR is centered on experiencing fully virtual worlds. As a result, people lose touch with reality. In a room-scale experience, like the HTC Vive, they can move—until they bump into a table or a wall because they are not familiar with the secure zone. Losing touch with your physical reality leads to a lot of problems. Right now, there is no solution for this using current VR technology.
- Why is it better than current AR?
- AR could accomplish the same thing as reality 2.0 in the long run. There is still quite a long way to go, however. Creating reality 2.0 in AR requires much more processing power and much better AR goggles than, for example, a Hololens. The Hololens has a very narrow field of view. This can and probably will be fixed in the near future. But you are still left with the problem of removing or replacing an object using AR. Replacing something with nothing requires a lot of optical trickery and essentially many of the things reality 2.0 does—like recreating room geometry and building 3D scenes in real time. Compared to a fully virtual world, however, your options are much more limited and the experience won’t be as seamless.
- A few years from now: Imagine using ultra-high-resolution goggles where your eyes can no longer make out pixels on the screen. These will get smaller and lighter and be hooked up to more computational power and better 3D engines. Eventually, you’ll swap out the goggles and wear something like contact lenses, a futuristic idea that some companies are already working on.
- Imagine a not-so-distant-future, where it’s totally natural to upgrade your perception of reality and the border between real and virtual begins to crumble.
_______
14. Deep Learning Achievements Over the Past Year (dec 2017, 3650 mots raccourcis à 740, blog.statsbot.co)
- Compilation faire par Head of Machine Learning Team à Mail.ru Group
- Great developments in text, voice, and computer vision technologies
- Text
- Google Neural Machine Translation : closing down the gap with humans in accuracy of the translation by 55–85%
- Negotiations : a bot developed by Facebook learned one of the real negotiation strategies — showing a fake interest in certain aspects of the deal, only to give up on them later and benefit from its real goals. It has been the first attempt to create such an interactive bot, and it was quite successful.
- Voice
- Employees of DeepMind reported about generating audio.
- Their network was trained end-to-end: text for the input, audio for the output.
- The researches got an excellent result as the difference compared to human has been reduced by 50%.
- This same model can be applied not only to speech, but also, for example, to creating music.
- Lip reading
- Google Deepmind, in collaboration with Oxford University, reported in the article, “Lip Reading Sentences in the Wild” on how their model, which had been trained on a television dataset, was able to surpass the professional lip reader from the BBC channel.
- Synthesizing Obama: synchronization of the lip movement from audio
- The University of Washington has done a serious job of generating the lip movements of former US President Obama. The choice fell on him due to the huge number of his performance recordings online (17 hours of HD video).
- Employees of DeepMind reported about generating audio.
- Computer vision
- Better Optical Character Recognition for Google Maps and Street View (applied to 80 billion photos.)
- Visual reasoning
- it's about solving questions using a photo. For example: “Is there a same size rubber thing in the picture as a yellow metal cylinder?” The question is truly nontrivial, and until recently, the problem was solved with an accuracy of only 68.5%
- the breakthrough was achieved by the team from Deepmind: on the CLEVR dataset they reached a super-human accuracy of 95.5%.
- Generating code behind an interface according to a screenshot from the interface designer.
- The authors claim that they reached 77% accuracy
- Teaching a machine to draw
- Generative Adversarial Networks (GANs) (often, this idea is used to work with images)
- The idea is in the competition of two networks — the generator and the discriminator. The first network creates a picture, and the second one tries to understand whether the picture is real or generated.
- Changing face age with GANs
- Having trained the engine on the IMDB (Internet Movie Database) dataset with a known age of actors, the researchers were given the opportunity to change the face age of the person.
- Generating professional photos
- GAN was trained on a professional photo dataset: the generator is trying to improve bad photos (professionally shot and degraded with the help of special filters), and the discriminator — to distinguish “improved” photos and real professional ones.
- Synthesization of an image from a text description
- Transfiguring objects or styling
- Permet par exemple de changer le style d'une photo (passer de jour à nuit, d'été à hiver, de cheval à zèbre, de photo à dessin, etc. (voir les photos sur l'article)
- Adversarial-attacks
- adding special noise to a picture so that the picture with noise for the human eye is practically unchanged, while the model goes crazy and predicts a completely different thing. (si au départ on voit par ex une banane et l'IA aussi, juste en faisant qq modifs, là on ne voit pas la différence, l'IA voit qqchose qui n'a rien à voir)
- there's an example when adding special glasses to a face allow you to deceive the face recognition system and “pass yourself off as another person.”
- Reinforcement learning
- The essence of the approach is to learn the successful behavior of the agent in an environment that gives a reward through experience — just as people learn throughout their lives.
- RL is actively used in games, robots, and system management (traffic, for example).
- Of course, everyone has heard about AlphaGo’s victories in the game over the best professionals.
- Learning robots :
- OpenAI has been actively studying an agent’s training by humans in a virtual environment, which is safer for experiments than in real life.
- In one of the studies, the team showed that one-shot learning is possible: a person shows in VR how to perform a certain task, and one demonstration is enough for the algorithm to learn it and then reproduce it in real conditions.
- Learning on human preferences :
- Here is the work of OpenAI and DeepMind on the same topic. The bottom line is that an agent has a task, the algorithm provides two possible solutions for the human and indicates which one is better.
- Movement in complex environments
- Researchers managed to to teach agents (body emulators - bonhomme bâton) to perform complex actions by constructing a complex environment with obstacles and with a simple reward for progress in movement. (voir la vidéo incroyable de ce le bonhomme bâton parvient à apprendre à faire de lui-même)
______
15. The evolution of machine learning (août 2017, 1330 mots raccourcis à 225, techcrunch.com)
- Deep learning is a subcategory of machine learning algorithms that use multi-layered artificial neural networks to learn complex relationships between inputs and outputs. The more layers in the neural network, the more complexity it can capture.
- Traditional statistical machine learning algorithms (i.e. ones that do not use deep neural nets) have a more limited capacity to capture information about training data.
- But these more basic machine learning algorithms work well enough for many applications, making the additional complexity of deep learning models often superfluous.
- Despite the focus on deep learning at the big tech company AI research labs, most applications of machine learning at these same companies do not rely on neural networks and instead use traditional machine learning models.
- These are the models behind, among other services tech companies use, friend suggestions, ad targeting, user interest prediction, supply/demand simulation and search result ranking.
- There are good reasons to use simpler models over deep learning. Deep neural networks are hard to train. They require more time and computational power (they usually require different hardware, specifically Graphics Processing Units - cartes graphiques utilisées pour les jeux vidéo). Getting deep learning to work is hard — it still requires extensive manual fiddling, involving a combination of intuition and trial and error.
- With traditional machine learning models, the time engineers spend on model training and tuning is relatively short — usually just a few hours.
______
16. AI Software Learns to Make AI Software (jan 2017, 570 mots raccourcis à 260, technologyreview.com)
- In one experiment, researchers at the Google Brain artificial intelligence research group created a software that could design a machine-learning system which surpassed software designed by humans.
- If self-starting AI techniques become practical, they could increase the pace at which machine-learning software is implemented across the economy, as for now companies must currently pay a premium for machine-learning experts, who are in short supply.
- Jeff Dean, who leads the Google Brain research group, mused last week that some of the work of such workers could be supplanted by software. He described what he termed “automated machine learning” as one of the most promising research avenues his team was exploring.
- “Currently the way you solve problems is you have expertise and data and computation,” said Google Brain's leader. “Can we eliminate the need for a lot of machine-learning expertise?”
- One set of experiments from Google’s DeepMind group suggests that what researchers are terming “learning to learn” could also help lessen the problem of machine-learning software needing to consume vast amounts of data on a specific task in order to perform it well.
- The researchers challenged their software to create learning systems for collections of multiple different, but related, problems, such as navigating mazes. It came up with designs that showed an ability to generalize, and pick up new tasks with less additional training than would be usual.
- Google Brain’s researchers created software that came up with software for image recognition systems that rivaled the best designed by humans.
______
17. Google’s AutoML lets you train custom machine learning models without having to code (jan 2017, 465 mots raccourcis à 30, techcrunch.com)
- Encore une info qui vient tordre le cou à l'idée obsessionnelle de L . Alexandre selon laquelle les GAFAMINBATXH vont régner en maître au détriment de toutes les autres entreprises et des consommateurs
- "Google’s new product AutoML lets you train custom machine learning models without having to code"
- "a new service that helps developers — including those with no machine learning (ML) expertise — build custom image recognition models. Google plans to expand this custom ML model builder to other areas, like speech, translation, video, natural language recognition, etc."
- "The basic idea here, Google says, is to allow virtually anybody to bring their images, upload them (and import their tags or create them in the app) and then have Google’s systems automatically create a customer machine learning model for them. "
- "The company says that Disney, for example, has used this system to make the search feature in its online store more robust because it can now find all the products that feature a likeness of Lightning McQueen and not just those where your favorite talking race car was tagged in the text description."
- "The whole process, from importing data to tagging it and training the model, is done through a drag and drop interface."
- "Google is opting for a system where it handles all of the hard work and trains and tunes your model for you."
- Certes les sceptiques diront qu'en chemin on donne toujours plus d'infos à Google, l'aidant gratuitement à devenir meilleur, et nous rendant encore plus dépendants que jamais de lui...peut-être, mais ça permet AUSSI à plus de monde d'appliquer l'IA à tous les domaines de l'économie.
- L'IA va devenir une "commodity", comme l'électricité.
- Et si Google ferme le robinet, Amazon, Facebook, etc. auront le leur d'ouvert.
- La concurrence devrait encore une fois profiter aux consommateurs qui jouiront de produits moins chers, meilleurs, plus intelligents, inédits, etc.
_________
Si cette veille vous a intéressé(e) et que vous ne recevez pas déjà nos emails, n'hésitez pas à renseigner votre adresse dans le champ plus bas de façon à recevoir une fois par semaine max les prochaines newsletters. Vous pouvez aussi renseigner l'email d'une ou un ami(e) !