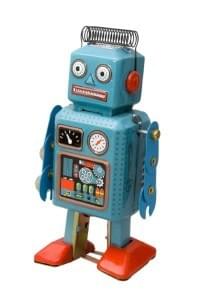
Parlons Futur : "Pas le temps de faire une veille approfondie pour anticiper le futur? Pas de soucis, je la fais pour vous! Voici ma sélection d'une dizaine de news du futur lues la semaine dernière et dont j'ai supprimé le blabla pour ne garder que la substance, pour vous faire gagner du temps."
Je ne m'étais pas présenté, je m'appelle Thomas Jestin, l'auteur de cette newsletter. Le jour, je suis le cofondateur et codirigeant de l'agence de communication digitale KRDS présente dans 6 pays entre Paris et l'Asie, et le reste du temps, je lis et écris sur le progrès technologique et ses implications sur la société, l'économie et plus largement le futur de l'aventure humaine. Vous pouvez retrouver tous mes écrits au-delà du thème de cette newsletter sur mon site www.thomasjestin.com.
Note : j'ai aussi résumé les articles à suivre et les commente dans un podcast, tapez simplement Parlons Futur dans votre appli de podcast préférée (si vous n'en avez pas je recommande Podcast Republic). Pratique pour se brieffer sur le futur "on the go", à vous de choisir la vitesse et de faire avance rapide pour aller aux articles de votre choix, j'ai détaillé les minutes en commentaires de l'épisode. C'est une première pour moi, je vous remercie par avance pour votre indulgence, et vos retours, conseils, remarques sont les bienvenus ! :)
Les articles à suivre que j'ai donc "raccourcis" et structurés en "bullet points":
- These People Never Existed. They Were Made by an AI. (30 oct 2017, futurism.com)
- Nvidia’s new AI creates disturbingly convincing fake videos (5 déc 2017, thenextweb.com)
- These Creepy Mini-Brains May Finally Crack Deadly Brain Cancer (12 déc 2017, singularityhub.com)
- Une recherche suggère que ce n'est pas la conscience qui dirige l'esprit humain (27 nov 2017, trustmyscience.com)
- AI Can Now Produce Better Art Than Humans. Here’s How. (8 juil 2017, futurism.com)
- New robots can see into their future (4 déc 2017, news.berkeley.edu)
- Google’s New AI Is Better at Creating AI Than the Company’s Engineers (19 mai 2017, futurism.com)
- Inside China's Vast New Experiment in Social Ranking (14 déc 2017, Wired, article de 6000 mots raccourcis ici à 1600 mots)
- The Business of Artificial Intelligence (2017, Harvard Business Review, article de 5000 mots raccourcis à 2000 mots)
- Computers are starting to reason like humans (14 juin 2017, www.sciencemag.org)
- AI's Implications for Productivity, Wages, and Employment (20 nov 2017, pcmag.com)
_____________
- These People Never Existed. They Were Made by an AI. (30 oct 2017, futurism.com)
- https://futurism.com/these-people-never-existed-they-were-made-by-an-ai/
- Chipmaker NVIDIA has developed an AI that produces highly detailed images of human-looking faces, but the people depicted don't actually exist. The system is the latest example of how AI is blurring the line between the "real" and the fabricated.
- https://youtu.be/XOxxPcy5Gr4?t=39
_______
- Nvidia’s new AI creates disturbingly convincing fake videos (5 déc 2017, thenextweb.com)
- https://thenextweb.com/artificial-intelligence/2017/12/04/nvidias-new-ai-creates-disturbingly-convincing-fake-videos/
- Researchers from Nvidia have created an image translation AI that will almost certainly have you second-guessing everything you see online. The system can change day into night, winter into summer, and house cats into cheetahs with minimal training materials
- The AI does a surprisingly decent job of changing day into night, winter into summer, and house cats into cheetahs (and vice versa). Best (or worst?) of all, the AI does it all with much less training than existing systems.
- https://www.youtube.com/watch?v=9VC0c3pndbI
_______
- These Creepy Mini-Brains May Finally Crack Deadly Brain Cancer (12 déc 2017, singularityhub.com)
- https://singularityhub.com/2017/12/12/these-creepy-mini-brains-may-finally-crack-deadly-brain-cancer/
- Made from cells directly taken from human donors, Brain organoids, charmingly dubbed “mini-brains” and “brain balls”, are tiny clumps of cells roughly mimic how a human brain develops. Under a combination of growth chemicals and nurturing care, they expand to a few centimeters in diameter as their neurons extend their branches and hook up basic neural circuits.
- Brain balls are as close as scientists can get to recreating brain development in a dish, where the process can be studied and tinkered with. To most neuroscientists, they could be the key to finally cracking what goes awry in autism, schizophrenia, and a myriad of other brain developmental disorders.
- As it happens, tumor stem cells are also tough to grow in the lab. So when scientists carefully prepare the cells to transplant into mice, they inadvertently miss one of the most crucial populations. The result is that glioblastomas are mysteriously tame after transplantation: they’re not nearly as aggressive as their original source. In other words, scientists don’t really have a good way to study glioblastomas. Lacking a suitable model makes testing potential new drugs or other therapies extremely difficult.
- Could these quasi-human brains replace mice brains? he wondered.
- In roughly six weeks, his team grew mini-brains roughly the same level of development as a 20-week-old human fetus. When placed together with glioblastoma stem cells from patients in a dish, the cancer cells readily clamp onto the mini-brains. Within 24 hours, they begin driving their tentacles deeper into the brain-like tissue in a pattern “that looks 100 percent like what happens in the patient’s own brain,” says Fine.
- The plan is to “make hundreds of brain organoids for any given patient and use them to screen for drugs that can shrink that patient’s tumor,” he says.
- Une recherche suggère que ce n'est pas la conscience qui dirige l'esprit humain (27 nov 2017, trustmyscience.com)
- http://trustmyscience.com/la-conscience-ne-dirigerait-pas-l-esprit-humain/
- La plupart des experts dans le domaine, pensent que la conscience peut être divisée en deux parties :
- l’expérience de la conscience (ou la conscience personnelle)
- et le contenu de la conscience, incluant des éléments tels que les pensées, les croyances, les sensations, les perceptions, les intentions, les souvenirs et les émotions.
- Il est facile de supposer que les contenus de la conscience soient en quelque sorte choisis, provoqués ou contrôlés par notre conscience personnelle : après tout, nos pensées concernant un sujet n’existent pas tant que nous n’y pensons pas.
- Mais une nouvelle recherche publiée dans Frontiers of Psychology soutient qu’il s’agit d’une erreur. L’étude suggère en effet que notre conscience personnelle ne crée pas réellement, qu’elle ne cause pas ou ne choisit pas nos croyances, nos sentiments ou nos perceptions. Au lieu de cela, les contenus de la conscience sont générés par des systèmes rapides, efficaces et non conscients de notre cerveau. Tout cela se passe sans aucune interférence de notre conscience personnelle, qui reste passive lors de ces processus.
- En gros, nous ne choisissons pas consciemment nos pensées ou nos sentiments, nous en prenons conscience.
- Les chercheurs soutiennent que le contenu de la conscience est un sous-ensemble des expériences, des émotions, des pensées et des croyances qui sont générées par des processus non-conscients dans le cerveau.
- Ce sous-ensemble prend la forme d’un récit personnel, qui est constamment mis à jour.
- En effet, le récit personnel existe parallèlement à notre conscience personnelle, mais celle-ci n’a aucune influence sur le premier.
- Le récit personnel est important car il fournit des informations à stocker dans la mémoire autobiographique (l’histoire que nous nous racontons, à propos de nous) et nous donne à nous, êtres humains, un moyen de communiquer aux autres les choses que nous avons perçues et expérimentées. Puis ceci, à son tour, permet de générer des stratégies de survie : par exemple, en apprenant à analyser et à prédire le comportement des autres. Les compétences interpersonnelles comme celle-ci soutiennent le développement des structures sociales et culturelles, qui ont favorisé la survie du genre humain depuis des millénaires.
- Si l’expérience de la conscience ne confère aucun avantage particulier, son but n’est pas clair pour les scientifiques. Mais en tant qu’accompagnement passif des processus non conscients, les chercheurs ne pensent pas que les phénomènes de la conscience personnelle aient forcément un but.
- Les conclusions de l’étude soulèvent également des questions sur les notions de libre arbitre et de responsabilité personnelle. Car si notre conscience personnelle ne contrôle pas le contenu du récit personnel qui reflète nos pensées, nos sentiments, nos émotions, nos actions et nos décisions, alors à quel point sommes-nous réellement responsables de ces éléments ?
- En réponse à cela, les chercheurs suggèrent que le libre arbitre et la responsabilité personnelle sont des notions qui ont été construites par la société. En tant que tels, ces éléments sont construits dans la manière dont nous voyons et nous nous comprenons nous-mêmes en tant qu’individus, et en tant qu’espèce. C’est pour cette raison qu’ils sont représentés dans les processus non-conscients qui donnent naissance à nos récits personnels, et dans la manière dont nous communiquons ces récits aux autres.
- Mais ce n’est pas pour autant que nous devons nous passer des notions quotidiennes importantes telles que le libre arbitre et la responsabilité personnelle. En fait, ils sont intégrés dans le fonctionnement de nos systèmes cérébraux non conscients, gardent un but puissant dans la société et ont un impact profond sur la façon dont nous nous comprenons nous-mêmes.
- AI Can Now Produce Better Art Than Humans. Here’s How. (8 juil 2017, futurism.com)
- https://futurism.com/ai-now-produce-better-art-humans-heres-how/
- Scientists have created an artificially intelligent system that is capable of producing cutting edge paintings that some consider to be better than works created by humans.
- The scientists changed the way AI usually produces art by having it only create works that did not fall into a preexistent category of painting
- After the paintings were produced, the scientists conducted a survey with members of the public in which they mixed the AI works with paintings produced by human artists. They found that the public preferred the works by AI, and thought they were more novel, complex, and inspiring.
- New robots can see into their future (4 déc 2017, news.berkeley.edu)
- http://news.berkeley.edu/2017/12/04/robots-see-into-their-future/
- UC Berkeley researchers have developed a robotic learning technology that enables robots to imagine the future of their actions so they can figure out how to manipulate objects they have never encountered before. In the future, this technology could help self-driving cars anticipate future events on the road and produce more intelligent robotic assistants in homes, but the initial prototype focuses on learning simple manual skills entirely from autonomous play.
- Using this technology, called visual foresight, the robots can predict what their cameras will see if they perform a particular sequence of movements. These robotic imaginations are still relatively simple for now – predictions made only several seconds into the future – but they are enough for the robot to figure out how to move objects around on a table without disturbing obstacles.
- Crucially, the robot can learn to perform these tasks without any help from humans or prior knowledge about physics, its environment or what the objects are. That’s because the visual imagination is learned entirely from scratch from unattended and unsupervised exploration, where the robot plays with objects on a table. After this play phase, the robot builds a predictive model of the world, and can use this model to manipulate new objects that it has not seen before.
- https://www.youtube.com/watch?v=Li_vZVpiFSA
- Computers are starting to reason like humans (14 juin 2017, www.sciencemag.org)
- http://www.sciencemag.org/news/2017/06/computers-are-starting-reason-humans
- relational reasoning is an important component of higher thought that has been difficult for artificial intelligence (AI) to master.
- Now, researchers at Google’s DeepMind have developed a simple algorithm to handle such reasoning—and it has already beaten humans at a complex image comprehension test.
- Humans are generally pretty good at relational reasoning, a kind of thinking that uses logic to connect and compare places, sequences, and other entities.
- But the two main types of AI—statistical and symbolic—have been slow to develop similar capacities.
- Statistical AI, or machine learning, is great at pattern recognition, but not at using logic.
- And symbolic AI can reason about relationships using predetermined rules, but it’s not great at learning on the fly.
- The new study proposes a way to bridge the gap: an artificial neural network for relational reasoning : “We’re explicitly forcing the network to discover the relationships that exist between the objects,”
- The AI was tasked to answer questions about relationships between objects in a single image, such as cubes, balls, and cylinders.
- For example: “There is an object in front of the blue thing; does it have the same shape as the tiny cyan thing that is to the right of the gray metal ball?”
- Humans scored a respectable 92%. The AI was correct 96% of the time, a superhuman score
- The DeepMind team also tried its neural net on a language-based task: on so-called inference questions ilke: “Lily is a Swan. Lily is white. Greg is a swan. What color is Greg?” (white), the relation network scored 98%, whereas best AIs until then scored about 45%.
- Google’s New AI Is Better at Creating AI Than the Company’s Engineers (19 mai 2017, futurism.com)
- https://futurism.com/googles-new-ai-is-better-at-creating-ai-than-the-companys-engineers/
- Google shared details of its AutoML project, an artificial intelligence that can assist in the creation of other AIs. By automating some of the complicated process, AutoML could make machine learning more accessible to non-experts.
- So far, they have used the AutoML tech to design networks for :
- image recognition tasks : the system matched Google’s experts.
- and speech recognition tasks. : it exceeded them, designing better architectures than the humans were able to create.
- AI that can supplement human efforts to develop better machine learning technologies could democratize the field as the relatively few experts wouldn’t be stretched so thin. “If we succeed, we think this can inspire new types of neural nets and make it possible for non-experts to create neural nets tailored to their particular needs, allowing machine learning to have a greater impact to everyone,” according to Google’s blog post.
- AI's Implications for Productivity, Wages, and Employment (20 nov 2017, pcmag.com)
- http://sea.pcmag.com/feature/18333/ais-implications-for-productivity-wages-and-employment
- At a recent MIT conference on AI and the Future of Work a number of top economists talked about concerns that AI would lead to fewer jobs, or at least fewer good jobs, as well as debated the impact technology is having a productivity.
- Particularly interesting was Erik Brynjolfsson, Director of the MIT Initiative on the Digital Economy
- Yealy labor productivity growth in business sector in the US was higher over 1947-1973 (3.2%) than in any period since then (2.7% over 2000-2007 was the second highest period)!
- Brynjolfsson gave four possible reasons that he believes may account for the productivity paradox.
- We may have false hopes, he said, and it may be the case that new technology simply won’t prove to provide significant productivity gains.
- It may also be that productivity is mismeasured, meaning that we are not tracking the real benefits of technology.
- The productivity improvements could be affecting only a few people, industries, or organizations, and not the general public.
- Or—and this is the explanation he believes makes the most sense—that the technology improvements are real, but that because organizations take a long time to restructure themselves, it in turn takes a long time for the benefits of advances in technology to emerge.
- In general, he said, optimists are extrapolating future impacts of current technologies, while pessimists are extrapolating future trends from recent GDP and productivity data.
- Brynjolfsson said AI is a General-Purpose Technology (GPT) and noted that such technologies may actually lower stated productivity up front as companies invest in these without seeing a return, which comes later. He said that the statistics we use are not predictions of the future, but rather "a measure of our ignorance.”
- In general, he said GPTs require time-consuming complementary innovation and investment, and that to keep up with accelerating technology in order to realize the benefits of AI, we will probably need to reinvent our organizations, institutions, and metrics.
- For comparison, he talked about how, despite the invention of the electric engine and light bulb, we didn't see much productivity gain between 1890-1920.
- Factories often replaced steam engines with electric engines, but the basic design of a factory—designed around a big central power source—didn’t change. In fact it would take 20-30 years until a new kind of factory—one that used small electric motors distributed throughout the factory—became popular.
- This led to changes in order and production, with the introduction of assembly lines, which in turn produced a big improvement in the 1920's. That was followed by a period of "secular stagnation"—the phrase applied to productivity numbers in recent years—and later, another boom.
- Brynjolfsson argued that one way to think about this is that AI and the investments people are making in organizational changes may be unmeasured intangible capital. For instance, he said, the productivity statistics will show time and money being spent on self-driving cars, but because they aren't sold yet, this won't register as having created productivity. As a result, he said, though we might be seeing lower productivity now, we will see higher productivity numbers in the future.
- Northwestern University Professor Joel Mokyr said that the boundaries between work and leisure are fuzzy, and noted that 25 percent of Americans do some volunteer work. He said the biggest improvement has been in leisure, and referenced work by some economists that suggests the decline in labor force participation has come in part because prime-age males are hooked on video games.
- Asked about what we should do to make things better for people, Brynjolfsson said most economists would put education at the top of the list, followed by doing more to encourage entrepreneurship. "Too often, the government is trying to protect the past from the future," he said. He also encouraged a strengthening of the safety net, and in particular the earned income tax credit.
_______
- Inside China's Vast New Experiment in Social Ranking (14 déc 2017, Wired)
- https://www.wired.com/story/age-of-social-credit/
- Owned by Ant Financial, an affiliate of the massive Alibaba corporation, Alipay is sometimes called a super app. Its main competitor, WeChat, belongs to the social and gaming giant Tencent. Alipay and WeChat are less like individual apps than entire ecosystems. Whenever Liu opened Alipay on his phone, he saw a neat grid of icons that vaguely resembled the home screen on his Samsung. Some of the icons were themselves full-blown third-party apps. If he wanted to, he could access Airbnb, Uber, or Uber’s Chinese rival Didi, entirely from inside Alipay. It was as if Amazon had swallowed eBay, Apple News, Groupon, American Express, Citibank, and YouTube—and could siphon up data from all of them.
- One day a new icon appeared on Liu’s Alipay home screen. It was labeled Zhima Credit (or Sesame Credit). The name, like that of Alipay’s parent company, evoked the story of Ali Baba and the 40 thieves, in which the words open sesame magically unseal a cave full of treasure. When Liu touched the icon, he was greeted by an image of the Earth. “Zhima Credit is the embodiment of personal credit,” the text underneath read. “It uses big data to conduct an objective assessment. The higher the score, the better your credit.”
- During the past 30 years, by contrast, China has grown to become the world’s second largest economy without much of a functioning credit system at all.
- Still, efforts to establish a reliable credit system foundered because China lacked a third-party credit scoring entity.
- What it did have by the end of 2011 were 356 million smartphone users. That year, Ant Financial launched a version of Alipay with a built-in scanner for reading QR codes
- WeChat Pay, which launched in 2013, has a similar built-in scanner.
- Codes started showing up on graves (scan to learn more about the deceased) and the shirts of waiters (scan to tip). Beggars printed out QR codes and set them out on the street. The codes linked the online and offline realms on a scale not seen anywhere else in the world. That first year with the QR scanner, Alipay mobile payments reached nearly $70 billion.
- The executives realized that they could use the data-collecting powers of Alipay to calculate a credit score based on an individual’s activities. “It was a very natural process,” says You Xi, a Chinese business reporter who detailed this pivotal meeting in a recent book, Ant Financial. “If you have payment data, you can assess the credit of a person.” And so the tech company began the process of creating a score that would be “credit for everything in your life,”
- Coincidentally or not, in 2014 the Chinese government announced it was developing what it called a system of “social credit.” In 2014, the State Council, China’s governing cabinet, publicly called for the establishment of a nationwide tracking system to rate the reputations of individuals, businesses, and even government officials. The aim is for every Chinese citizen to be trailed by a file compiling data from public and private sources by 2020, and for those files to be searchable by fingerprints and other biometric characteristics. The State Council calls it a “credit system that covers the whole society.”
- For the Chinese Communist Party, social credit is an attempt at a softer, more invisible authoritarianism. The goal is to nudge people toward behaviors ranging from energy conservation to obedience to the Party.
- the government wants to preempt instability that might threaten the Party.
- To aid in the task, the government has enlisted Baidu, a big tech company, to help develop the social credit database by the 2020 deadline.
- In 2015 Ant Financial was one of eight tech companies granted approval from the People’s Bank of China to develop their own private credit scoring platforms. Zhima Credit appeared in the Alipay app shortly after that. The service tracks your behavior on the app to arrive at a score between 350 and 950, and offers perks and rewards to those with good scores. Zhima Credit’s algorithm considers not only whether you repay your bills but also what you buy, what degrees you hold, and the scores of your friends.
- Ant Financial executives talked publicly about how a data-driven approach would open up the financial system to people who had been locked out, like students and rural Chinese. For the more than 200 million Alipay users who have opted in to Zhima Credit, the sell is clear: Your data will magically open doors for you.
- Participating in Zhima Credit is voluntary, and it’s unclear whether or how signing up for it could affect an individual’s rating in the government system.
- “Zhima Credit is dedicated to creating trust in a commercial setting and independent of any government-initiated social credit system,” the statement reads.
- Ant Financial did state, however, in a 2015 press release that the company plans “to help build a social integrity system.” And the company has already cooperated with the Chinese government in one important way: It has integrated a blacklist of more than 6 million people who have defaulted on court fines into Zhima Credit’s database.
- The State Council has signaled that under the national social credit system people will be penalized for the crime of spreading online rumors, among other offenses, and that those deemed “seriously untrustworthy” can expect to receive substandard services.
- Because of my middling score, however, I had to pay a $30 deposit before I could scan my first bike. Nor could I get deposit-free hotel stays or GoPro rentals, or free umbrella rentals. I belonged to the digital underclass.
- In China, anxiety about pianzi, or swindlers, runs deep. How do I know you’re not a pianzi? is a question people often ask when salespeople call on the phone or repairmen show up at the door.
- As economic reforms in the 1980s led millions of people to leave their villages and migrate to cities, the work unit system fell apart. Migration also had a secondary effect: Cities filled up with strangers and pianzi.
- a car rental company, allows people with credit scores over 650 to rent a car without a deposit. In exchange for this vetting, Shenzhou Zuche shares data, so that if a Zhima Credit user crashes one of the rental company’s cars and refuses to pay up, that detail is fed back into his or her credit score. For a while people with scores over 750 could even skip the security check line at Beijing Capital Airport.
- After starting at 600 out of a possible 950 points, he had reached 722, a score that entitled him to favorable terms on loans and apartment rentals, as well as showcasing on several dating apps should he and his wife ever split up. With a few dozen more points, he could get a streamlined visa to Luxembourg
- In June 2015, as 9.4 million Chinese teenagers took the grueling national college entrance examination, Hu Tao, the Zhima Credit general manager, told reporters that Ant Financial hoped to obtain a list of students who cheated, so that the fraud could become a blight on their Zhima Credit records. “There should be consequences for dishonest behavior,” she avowed.
- The algorithm behind my Zhima Credit score is a corporate secret. Ant Financial officially lists five broad categories of information that feed into the score, but the company provides only the barest of details about how these ingredients are cooked together.
- A category called Connections considers the credit of my contacts in Alipay’s social network.
- Characteristics takes into consideration what kind of car I drive, where I work, and where I went to school.
- A category called Behavior, meanwhile, scrutinizes the nuances of my consumer life, zeroing in on actions that purportedly correlate with good credit. Shortly after Zhima Credit’s launch, the company’s technology director, Li Yingyun, told the Chinese magazine Caixin that spending behavior like buying diapers, say, could boost one’s score, while playing videogames for hours on end could lower it.
- One day last May, Liu Hu, a 42-year-old journalist, opened a travel app to book a flight. But when he entered his name and national ID number, the app informed him that the transaction wouldn’t go through because he was on the Supreme People’s Court blacklist. This list—literally, the List of Dishonest People—is the same one that is integrated into Zhima Credit. In 2015 Liu had been sued for defamation by the subject of a story he’d written, and a court had ordered him to pay $1,350. He paid the fine, and even photographed the bank transfer slip and messaged the photo to the judge in the case. Perplexed as to why he was still on the list, he contacted the judge and learned that, while transferring his fine, he had entered the wrong account number.
- Although Liu hadn’t signed up for Zhima Credit, the blacklist caught up with him in other ways. He became, effectively, a second-class citizen. He was banned from most forms of travel; he could only book the lowest classes of seat on the slowest trains. He could not buy certain consumer goods or stay at luxury hotels, and he was ineligible for large bank loans. Worse still, the blacklist was public.
- Now I had two tracking systems scoring me, on opposite sides of the globe. But these were only the scores that I knew about. Most Americans have dozens of scores, many of them drawn from behavioral and demographic metrics similar to those used by Zhima Credit, and most of them held by companies that give us no chance to opt out.
- In 2012, Facebook patented a method of credit assessment that could consider the credit scores of people in your network. The patent describes a tool that arrives at an average credit score for your friends and rejects a loan application if that average is below a certain minimum. The company could still decide to get into the credit business itself, though
- The Business of Artificial Intelligence (2017, Harvard Business Review)
- https://hbr.org/cover-story/2017/07/the-business-of-artificial-intelligence
- by Erik Brynjolfsson and Andrew McAfee, authors of : Machine, Platform, Crowd: Harnessing Our Digital Future (2017) and the New York Times best seller The Second Machine Age: Work, Progress, and Prosperity in a Time of Brilliant Technologies (2014).
- The most important general-purpose technology of our era is artificial intelligence, particularly machine learning (ML) — that is, the machine’s ability to keep improving its performance without humans having to explain exactly how to accomplish all the tasks it’s given.
- Big deal because :
- First, we humans know more than we can tell: We can’t explain exactly how we’re able to do a lot of things — from recognizing a face to making a smart move in the ancient Asian strategy game of Go. Prior to ML, this inability to articulate our own knowledge meant that we couldn’t automate many tasks. Now we can.
- Second, ML systems are often excellent learners. They can achieve superhuman performance in a wide range of activities, including detecting fraud and diagnosing disease.
- The effects of AI will be magnified in the coming decade, as manufacturing, retailing, transportation, finance, health care, law, advertising, insurance, entertainment, education, and virtually every other industry transform their core processes and business models to take advantage of machine learning.
- The bottleneck now is in management, implementation, and business imagination.
- The biggest advances have been in two broad areas:
- perception, where most practical advances have been made in relation to speech
- speech recognition's error rate, once 8.5%, has dropped to 4.9%. What’s striking is that this substantial improvement has come not over the past 10 years but just since the summer of 2016.
- The error rate for recognizing images from a large database called ImageNet, with several million photographs of common, obscure, or downright weird images, fell from higher than 30% in 2010 to about 4% in 2016 for the best systems (humans have a 5% error rate)
- cognition and problem solving.
- Machines have already beaten the finest (human) players of poker and Go — achievements that experts had predicted would take at least another decade.
- Intelligent agents are being used by the cybersecurity company Deep Instinct to detect malware, and by PayPal to prevent money laundering.
- Dozens of companies are using ML to decide which trades to execute on Wall Street, and more and more credit decisions are made with its help.
- Amazon employs ML to optimize inventory and improve product recommendations to customers.
- Infinite Analytics developed one ML system :
- to predict whether a user would click on a particular ad, improving online ad placement for a global consumer packaged goods company,
- increased advertising ROI 3X
- and another ML system to improve customers’ search and discovery process at a Brazilian online retailer.
- resulted in a $125 million increase in annual revenue.
- to predict whether a user would click on a particular ad, improving online ad placement for a global consumer packaged goods company,
- perception, where most practical advances have been made in relation to speech
- Aptonomy and Sanbot, makers respectively of drones and robots, are using improved vision systems to automate much of the work of security guards
- The software company Affectiva, among others, is using them to recognize emotions such as joy, surprise, and anger in focus groups
- Enlitic is one of several deep-learning startups that use them to scan medical images to help diagnose cancer.
- But ML systems are trained to do specific tasks, and typically their knowledge does not generalize. The fallacy that a computer’s narrow understanding implies broader understanding is perhaps the biggest source of confusion, and exaggerated claims, about AI’s progress. We are far from machines that exhibit general intelligence across diverse domains.
- The most important thing to understand about ML is that it represents a fundamentally different approach to creating software: The machine learns from examples, rather than being explicitly programmed for a particular outcome. This is an important break from previous practice. For most of the past 50 years, advances in information technology and its applications have focused on codifying existing knowledge and procedures and embedding them in machines.
- This approach has a fundamental weakness: Much of the knowledge we all have is tacit, meaning that we can’t fully explain it. It’s nearly impossible for us to write down instructions that would enable another person to learn how to ride a bike or to recognize a friend’s face.
- Machine learning is overcoming those limits. In this second wave of the second machine age, machines built by humans are learning from examples and using structured feedback to solve on their own problems
- Artificial intelligence and machine learning come in many flavors, but most of the successes in recent years have been in one category: supervised learning systems, in which the machine is given lots of examples of the correct answer to a particular problem. This process almost always involves mapping from a set of inputs, X, to a set of outputs, Y.
- For instance, the inputs might be pictures of various animals, and the correct outputs might be labels for those animals: dog, cat, horse. The inputs could also be waveforms from a sound recording and the outputs could be words: “yes,” “no,” “hello,” “good-bye.”
- The algorithms that have driven much of this success depend on an approach called deep learning, which uses neural networks. Deep learning algorithms have a significant advantage over earlier generations of ML algorithms: They can make better use of much larger data sets. The old systems would improve as the number of examples in the training data grew, but only up to a point, after which additional data didn’t lead to better predictions. According to Andrew Ng, one of the giants of the field, deep neural nets don’t seem to level off in this way: More data leads to better and better predictions.
- Any situation in which you have a lot of data on behavior and are trying to predict an outcome is a potential application for supervised learning systems.
- JPMorgan Chase introduced a system for reviewing commercial loan contracts; work that used to take loan officers 360,000 hours can now be done in a few seconds.
- Unsupervised learning systems seek to learn on their own. We humans are excellent unsupervised learners: We pick up most of our knowledge of the world (such as how to recognize a tree) with little or no labeled data. But it is exceedingly difficult to develop a successful machine learning system that works this way.
- These machines could look at complex problems in fresh ways to help us discover patterns — in the spread of diseases, in price moves across securities in a market, in customers’ purchase behaviors, and so on — that we are currently unaware of. Such possibilities lead Yann LeCun, the head of AI research at Facebook and a professor at NYU, to compare supervised learning systems to the frosting on the cake and unsupervised learning to the cake itself.
- Another small but growing area within the field is reinforcement learning. This approach is embedded in systems that have mastered Atari video games and board games like Go. It is also helping to optimize data center power usage and to develop trading strategies for the stock market.
- In reinforcement learning systems the programmer specifies the current state of the system and the goal, lists allowable actions, and describes the elements of the environment that constrain the outcomes for each of those actions. Using the allowable actions, the system has to figure out how to get as close to the goal as possible. These systems work well when humans can specify the goal but not necessarily how to get there.
- There are three pieces of good news for organizations looking to put ML to use today.
- 1. First, AI skills are spreading quickly. The world still has not nearly enough data scientists and machine learning experts, but the demand for them is being met by online educational resources as well as by universities. The best of these, including Udacity, Coursera, and fast.ai, do much more than teach introductory concepts; they can actually get smart, motivated students to the point of being able to create industrial-grade ML deployments.
- 2. The second welcome development is that the necessary algorithms and hardware for modern AI can be bought or rented as needed. Google, Amazon, Microsoft, Salesforce, and other companies are making powerful ML infrastructure available via the cloud. The cutthroat competition among these rivals means that companies that want to experiment with or deploy ML will see more and more capabilities available at ever-lower prices over time.
- 3. The final piece of good news, and probably the most underappreciated, is that you may not need all that much data to start making productive use of ML. The performance of most machine learning systems improves as they’re given more data to work with, so it seems logical to conclude that the company with the most data will win. That might be the case if “win” means “dominate the global market for a single application such as ad targeting or speech recognition.” But if success is defined instead as significantly improving performance, then sufficient data is often surprisingly easy to obtain.
- After 1,000 training cycles, the salespeople had increased their effectiveness by 54% and were able to serve twice as many customers at a time.
- Machine learning is driving changes at 3 levels:
- tasks and occupations
- An example of task-and-occupation redesign is the use of machine vision systems to identify potential cancer cells — freeing up radiologists to focus on truly critical cases, to communicate with patients, and to coordinate with other physicians.
- business processes,
- An example of process redesign is the reinvention of the workflow and layout of Amazon fulfillment centers after the introduction of robots and optimization algorithms based on machine learning.
- and business models.
- Similarly, business models need to be rethought to take advantage of ML systems that can intelligently recommend music or movies in a personalized way. Instead of selling songs à la carte on the basis of consumer choices, a better model might offer a subscription to a personalized station that predicted and played music a particular customer would like, even if the person had never heard it before.
- tasks and occupations
- Note that machine learning systems hardly ever replace the entire job, process, or business model. Most often they complement human activities, which can make their work ever more valuable. The most effective rule for the new division of labor is rarely, if ever, “give all tasks to the machine.” Instead, if the successful completion of a process requires 10 steps, one or two of them may become automated while the rest become more valuable for humans to do.
- machine learning systems often have low “interpretability,” meaning that humans have difficulty figuring out how the systems reached their decisions.
- three risks.
- First, the machines may have hidden biases, derived not from any intent of the designer but from the data provided to train the system. For instance, if a system learns which job applicants to accept for an interview by using a data set of decisions made by human recruiters in the past, it may inadvertently learn to perpetuate their racial, gender, ethnic, or other biases. Moreover, these biases may not appear as an explicit rule but, rather, be embedded in subtle interactions among the thousands of factors considered.
- A second risk is that, unlike traditional systems built on explicit logic rules, neural network systems deal with statistical truths rather than literal truths. That can make it difficult, if not impossible, to prove with complete certainty that the system will work in all cases — especially in situations that weren’t represented in the training data. Lack of verifiability can be a concern in mission-critical applications, such as controlling a nuclear power plant, or when life-or-death decisions are involved.
- Third, when the ML system does make errors, as it almost inevitably will, diagnosing and correcting exactly what’s going wrong can be difficult. The underlying structure that led to the solution can be unimaginably complex, and the solution may be far from optimal if the conditions under which the system was trained change.
- We sometimes hear “Artificial intelligence will never be good at assessing emotional, crafty, sly, inconsistent human beings — it’s too rigid and impersonal for that.” We don’t agree.
- ML systems like those at Affectiva are already at or beyond human-level performance in discerning a person’s emotional state on the basis of tone of voice or facial expression.
- Other systems can infer when even the world’s best poker players are bluffing well enough to beat them at the amazingly complex game Heads-up No-Limit Texas Hold’em.
- Reading people accurately is subtle work, but it’s not magic. It requires perception and cognition — exactly the areas in which ML is currently strong and getting stronger all the time.
- In 2014 the TED Conference and the XPrize Foundation announced an award for “the first artificial intelligence to come to this stage and give a TED Talk compelling enough to win a standing ovation from the audience.” We doubt the award will be claimed anytime soon.
- We think the biggest and most important opportunities for human smarts in this new age of superpowerful ML lie at the intersection of two areas: figuring out what problems to work on next, and persuading a lot of people to tackle them and go along with the solutions. This is a decent definition of leadership, which is becoming much more important in the second machine age.
- In our view, artificial intelligence, especially machine learning, is the most important general-purpose technology of our era.
________
Je liste aussi ici les derniers articles que j'ai pu écrire dans la presse:
- 5 approximations de Laurent Alexandre face au Parlement Européen (Journal du Net, déc 2017)
- Réactions à l'article "The impossibility of intelligence explosion" (nov 2017)
- Comment nous discuterons demain : télépathie, changement de voix, et autres bizarreries (Journal du Net, nov 2017)
- Réponse à Jacques Attali qui se demande si l'IA peut rivaliser avec l'intelligence humaine et jouer les artistes (oct 2017), JA qui m'a d'ailleurs répondu sur Twitter : "Tres intéressante réponse, parlons en?"
______________
Et enfin quelques uns de mes derniers tweets:
- Google chief scientist Fei-Fei Li: “All three winning teams of the ImageNet Challenge in the past three years have been largely composed of Chinese researchers, Chinese authors contributed 43 percent of all content in the top 100 AI journals in 2015
- "when the Association for the Advancement of AI discovered that their annual meeting overlapped with Chinese New Year this year, they rescheduled.”
- style transfer, computed in real time, amazing : https://www.youtube.com/watch?time_continue=246&v=UYZMyV6bqKo
- AI-generated fake videos will soon become ubiquitous and realistic enough to disqualify videos as evidence. Low-quality fakes are already cropping up
- Descript transforms speech to text, puts it into a Word document, allows you to edit the sound file as a writer would edit a Word document. When you cut out a word in the transcription, it cuts it out in the sound file, and soon it will be possible to add a words.
- New Google app Storyboard instantly turns videos into single-page comic layouts on your device.
- Musk just predicted that his cars will be able to fully drive themselves better than a human in less than two years, and 100 times better in three years.
- Amazing, find the Last Common Ancestor between any living thing and you, for instance LCA of us and bats lived 85M y ago, we share with bats a 27 million-greats grandparent (best experienced from a laptop)
- In 2016, classified information about the arsenal of cyberweaponry at the NSA got hacked and leaked , including cyber weapons actively in development, iand hen used by ransomware “WannaCry” that began striking organizations from universities in China to hospitals in England
- Total energy use of bitcoin is huge, estimated 31 terawatt-hours/year. More than 150 individual countries consume less energy/year. It is increasing its energy use every day by 450 gigawatt-hours, roughly the same amount of electricity the entire country of Haiti uses in a year.
- Never ever before has the world changed as it has from 1990 to 2015.
- see the amazing chart here : https://twitter.com/DinaPomeranz/status/938104123324616709
- scientists delivered ultrasonic waves across the skull to brain regions that control the eyes or the legs, and could pingpong monkeys’ gazes and move sheeps’ hind legs.
- transcranial magnetic stimulation is another non invasive brain stimulation technique, has 2 pitfalls 1 they skim across the surface of the brain, can mostly target circuits in the cortex, missing crucial structures linked to memory, motivation, & reward. 2 they’re hard to target
- https://singularityhub.com/2017/11/29/how-bursts-of-high-frequency-sound-can-flip-switches-in-the-brain/#sm.000144qcpf1cr9djgwp6snwloaem0
- M. Hanczyc says Life is sthg that 1. has a body 2. can metabolise and 3. can inherit information. 1+2 enable movment and replication, add 3, u get evlution. @tegmark says life is ability to maintain one's complexity and replicate. By both definition, inorganic life seems possible
- Beautiful : 9 Robot Animals Built From Nature’s Best-Kept Secrets
- If the UN is right, there will be more Nigerian newborns than Chinese ones by the late 2050s
- Demographers at the UN estimate that there will be 140.89m births in 2018. That is 61,000 fewer than in 2017. # births/year is expected to drift down for several more years, then rise slightly, before finally peaking in the late 2040s at 1.5% above the present level
- 8,000x more energy from the sun hits the surface of the Earth in a day than we consume as a human race. Exponential decline in photovoltaic solar energy costs should let us meet between 50% & 100% of the world’s energy production from solar (and other renewables) in the next 20 yrs
- In 2016 scientists reported the creation of a minimal synthetic bacterial genome, containing just 473 genes (fyi, we have 20k). Of those 473 genes, 149 were of “unknown function”, but leaving out any one of them was lethal.
- proteins frequently form large complexes that function together, muddying the one gene-codes-for-one protein relationship. It thus becomes necessary to probe the function of genes in a combinatorial fashion.
- Doing this for the 149 genes of unknown function in the synthetic minimal genome using classical tools is a daunting task. Doing this for the human genome is impossible. However, with new technologies, scientists can now interrogate the function of genes at a much greater scale.
- Voir tous mes tweets : https://twitter.com/thomasjestin
Le podcast :
_________________________________
Si cette veille vous a intéressé(e) et que vous ne recevez pas déjà nos emails, n'hésitez pas à renseigner votre adresse dans le champ plus bas de façon à recevoir une fois par semaine max les prochaines newsletters.